“Multitasking” AI Tool Extracts Cancer Data in Record Time
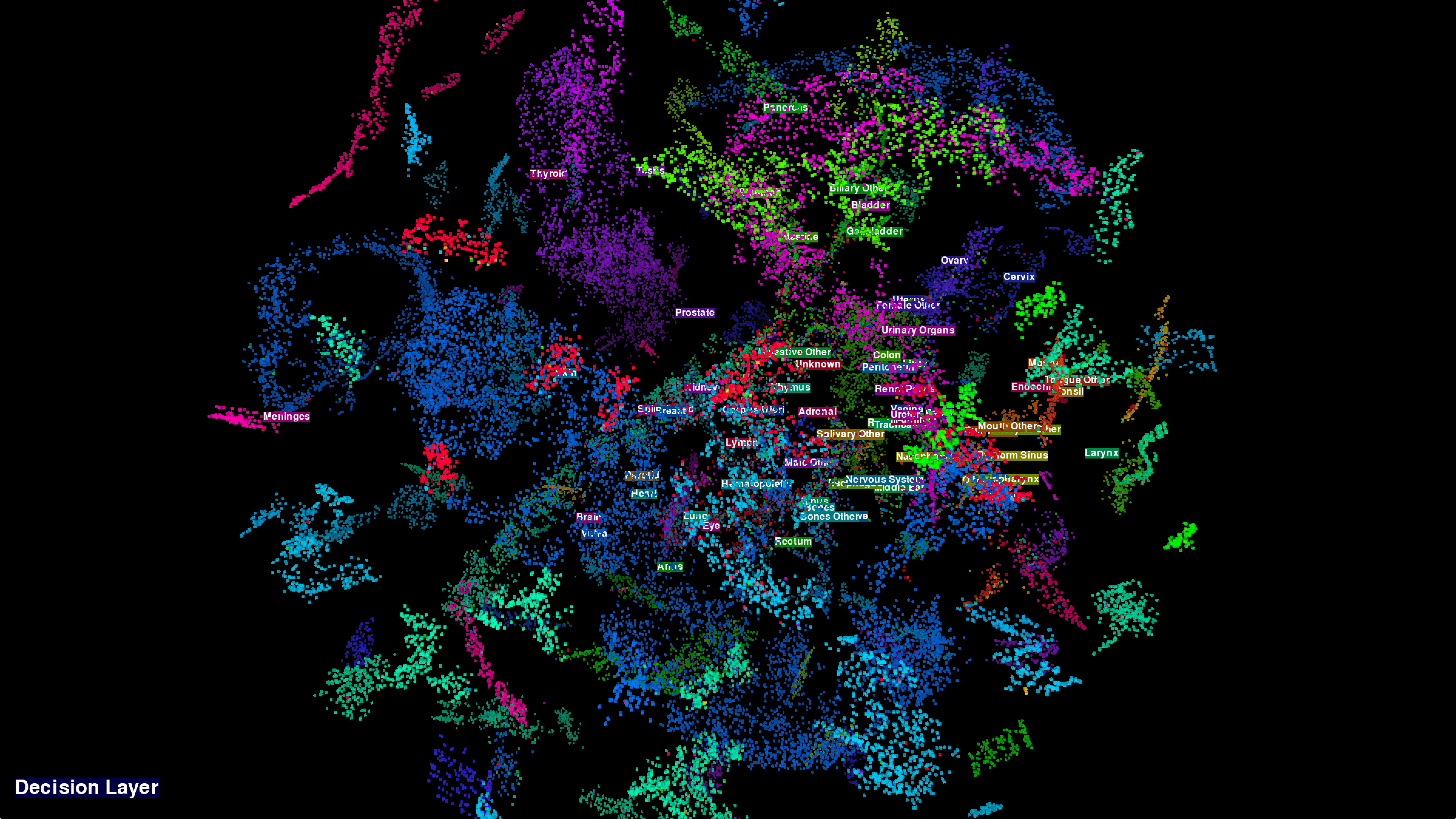
The Science
Digital cancer registries collect, manage, and store data on cancer patients. They help scientists identify trends in cancer diagnoses and treatment responses. This information can in turn help guide research funds and other resources. However, cancer pathology reports are complex. Human cancer researchers must analyze these reports to interpret variations in how they record information. To better leverage cancer data for research, scientists developed an artificial intelligence-based natural language processing tool. The tool will help researchers extract information from textual pathology reports.
The Impact
Researchers have developed a multitasking convolutional neural network (CNN) for cancer research. The CNN is the first of its kind for working with cancer pathology reports. The CNN is a deep learning model that learns to perform tasks, such as identifying key words in a body of text. It works by processing language as a two-dimensional numerical dataset. Researchers have previously used single-task CNN models to comb through pathology reports. However, each single-task model can extract only one characteristic from the range of information in the reports. Compared with the single-task CNN and conventional AI models, the new multitask CNN is much faster. It processed text reports in a fraction of the time as the single-task models. In addition, it more accurately classified each of the five cancer characteristics.
Summary
Population-level cancer surveillance is critical for monitoring the effectiveness of public health initiatives aimed at preventing, detecting, and treating cancer. To train and test the multitask CNNs with real health data, researchers used a secure data environment, more than 95,000 pathology reports from the Louisiana Tumor Registry, and the capabilities of the Oak Ridge Leadership Computing Facility, a Department of Energy Office of Science user facility. The researchers compared their multitask CNNs to three established AI models, including a single-task CNN. The researchers concluded that the multitask CNN offers superior classification accuracy for automated coding of cancer pathology documents. This finding was true across a wide range of cancers and across multiple information extraction tasks. The multitask CNN achieved this performance while needing training and inference time similar to that needed for a single task–specific model.
Contact
Gina Tourassi
Director, National Center for Computational Sciences, Oak Ridge National Laboratory
tourassig@ornl.gov
Funding
This work was supported in part by the Joint Design of Advanced Computing Solutions for Cancer (JDACS4C) program established by the Department of Energy and the National Cancer Institute (NCI) of the National Institutes of Health. This work was performed under the auspices of the Department of Energy by Argonne National Laboratory, Lawrence Livermore National Laboratory, Los Alamos National Laboratory, Oak Ridge National Laboratory, and the NCI’s Frederick National Laboratory for Cancer Research. This research used resources of the Oak Ridge Leadership Computing Facility at the Oak Ridge National Laboratory, which is supported by the Office of Science of the Department of Energy.
Publications
Alawad, M. et al., “Automatic extraction of cancer registry reportable information from free-text pathology reports using multitask convolutional neural networks.” Journal of the American Medical Informatics Association 27, 1 (2020). [DOI: 10.1093/jamia/ocz153]
Related Links
ORNL News: ORNL researchers develop ‘multitasking’ AI tool to extract cancer data in record time
Highlight Categories
Program: ASCR
Performer: DOE Laboratory , SC User Facilities , ASCR User Facilities , OLCF
Additional: Collaborations , Non-DOE Interagency Collaboration