MicroBooNE, Machine Learning, and Liquid Argon
The MicroBooNE experiment demonstrates the use of machine learning to interpret images made by a liquid-argon particle detector.
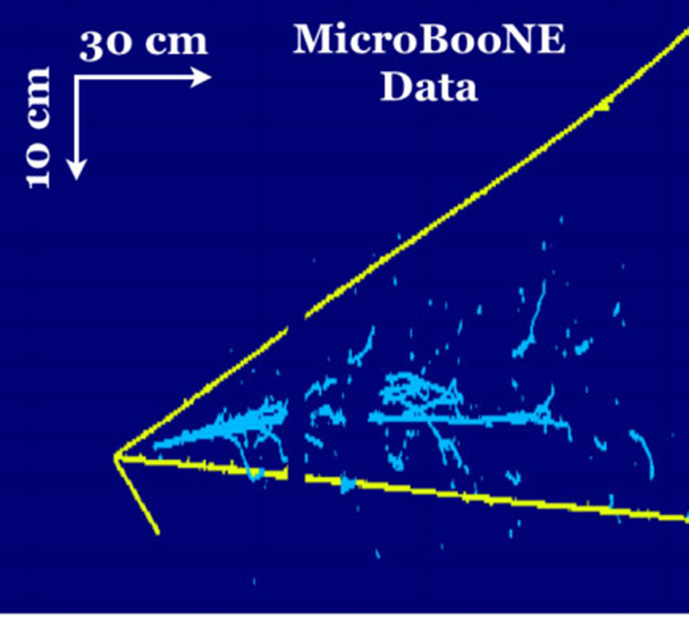
The Science
Decades ago, scientists studying tracks created by subatomic particles as they traveled through a detector identified the types of particles manually. The use of machine learning to interpret the image data is a technological leap forward. Now, the MicroBooNE neutrino experiment developed a type of machine learning algorithm—a convolutional neural network. The algorithm converts data from the tracks into information about the particles that generated them.
The Impact
The MicroBooNE team showed that its new machine-learning algorithm performs exceedingly well. It interprets simulated data and real data similarly. This algorithm is a valuable development for current and future neutrino experiments that use liquid-argon detector technology. It will help scientists learn about the properties of the neutrino and the fundamental laws of nature.
Summary
Researchers on the MicroBooNE neutrino experiment at Fermilab designed a type of machine learning algorithm, convolutional neural networks, to identify subatomic particles that interact in the MicroBooNE detector. The neural network sorted individual pixels coming from images made by a liquid-argon time projection chamber. The neural network, called U-ResNet, distinguished between two types of pixels: those that were a part of a track-like particle trajectory and those that were a part of a shower-like particle trajectory.
Track-like trajectories, made by particles such as a muon or proton, consist of a line with small curvature. Shower-like trajectories, produced by particles such as an electron or photon, are more complex topological features with many branching trajectories. This distinction is important because separating these types of topologies can be difficult for traditional algorithms. Not only that, shower-like shapes are produced when electrons and photons interact in the detector, and these two particles are often an important signal or background in physics analyses.
The team demonstrated that U-ResNet not only performs well, but also works in a similar fashion when presented with simulated data and real data. The latter is the first time this has been demonstrated for data from a liquid-argon neutrino detector. Convolutional neural networks are valuable to current and future neutrino experiments that will use liquid-argon neutrino detectors. The more demonstrations there are that these algorithms work on real detector data, the more confidence the community can have that convolutional neural networks will help elucidate the properties of the neutrino and the fundamental laws of nature.
Contact
Victor Genty
Columbia University
vg2337@columbia.edu
Kazuhiro Terao
SLAC National Accelerator Laboratory
kterao@slac.stanford.edu
Taritree Wongjirad
Tufts University
Taritree.Wongjirad@tufts.edu
Funding
This work was supported by the Department of Energy, Office of Science, Offices of High Energy Physics and Nuclear Physics; National Science Foundation; Swiss National Science Foundation; Science and Technology Facilities Council of the United Kingdom; and The Royal Society (United Kingdom). Additional support for the laser calibration system and cosmic ray tagger was provided by the Albert Einstein Center for Fundamental Physics.
Publications
C. Adams, M. Alrashed, R. An, et al. (MicroBooNE Collaboration), "A deep neural network for pixel-level electromagnetic particle identification in the MicroBooNE liquid argon time projection chamber.” Fermilab, FERMILAB-PUB-18-231-ND, Batavia, Illinois (2018). [arXiv: 1808.07269]
Related Links
Fermilab press release: MicroBooNE demonstrates use of convolutional neural networks on liquid-argon TPC data for first time
Highlight Categories
Performer: University , DOE Laboratory , SC User Facilities , HEP User Facilities , FermilabAC
Additional: Collaborations , Non-DOE Interagency Collaboration , International Collaboration